Vehicular mobility patterns and their applications to internet-of-vehicles: A comprehensive survey
Increasingly equipped with wireless interfaces, vehicles including driver-less vehicles make up a significant part of ubiquitous connection. As a consequence, not only do wireless networks grow quickly in terms of network scale, coverage and density, but become increasingly abundant of dynamics and variations such as fast-changing network topologies, channel states, and service demands.
Driven by big data, it is anticipated that learning techniques such as machine learning or deep learning will address many challenging communication and networking problems which have not been adequately addressed using optimization techniques and analyses.
The Internet-of Vehicles (IoV) is characterized by large-scale networks and continuous changes in the networks, and strong coupling in both the time and space domains. An application of learning techniques to the IoV is not trivial because of the network scale, continuous network changes, and strong network coupling.
Feature extraction is an effective way to reduce this input, facilitate the data-driven learning process, and improve efficiency. The vehicular mobility models are generated to share the features, rather than huge amounts of raw data, between the cities. This is expected to significantly reduce the bandwidth requirements, improve the generalization of learning results, and protect privacy.
This survey reviews the status quo of vehicular mobility models, with a particular emphasis on the recent results in the past decade. Existing vehicular mobility models are categorized into vehicular distribution, vehicular traffic, and driving behavior models.
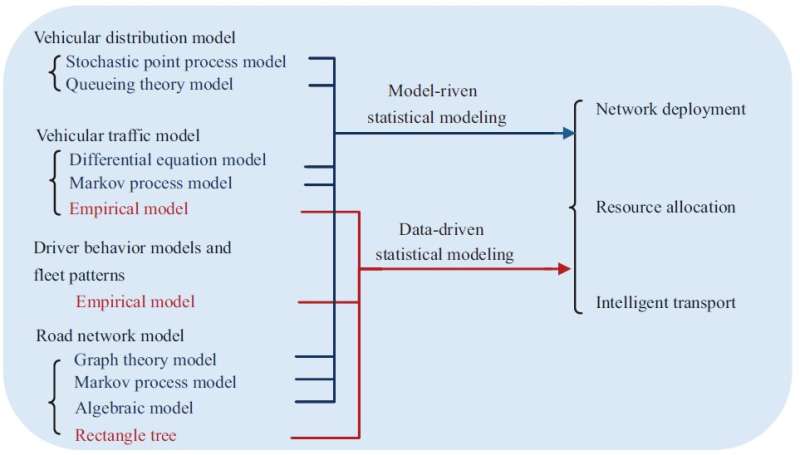
Such categorization has a particular emphasis on the random patterns of vehicles in space, traffic flow models aligned to road maps, and individuals’ driving behaviors (e.g., lane-changing and car-following). The different categories of the models are applied to various application scenarios, including underlying network connectivity analysis, off-line network optimization, online network functionality, and real-time autonomous driving.
We summarize the related application examples to cover a wide range of application scenarios in Intelligent Transport System (ITS). We assess the practicality of the models in the actual context of the ITS and discuss the potential performance of existing models, according to the characteristics of different application scenarios. Finally, several important research opportunities arise and deserve continuing research effort.
Qimei Cui et al, Vehicular mobility patterns and their applications to Internet-of-Vehicles: a comprehensive survey, Science China Information Sciences (2022). DOI: 10.1007/s11432-021-3487-x
Citation:
Vehicular mobility patterns and their applications to internet-of-vehicles: A comprehensive survey (2022, November 15)
retrieved 15 November 2022
from https://techxplore.com/news/2022-11-vehicular-mobility-patterns-applications-internet-of-vehicles.html
This document is subject to copyright. Apart from any fair dealing for the purpose of private study or research, no
part may be reproduced without the written permission. The content is provided for information purposes only.
For all the latest Technology News Click Here
For the latest news and updates, follow us on Google News.