A robot that can autonomously explore real-world environments
Roboticists have developed many advanced systems over the past decade or so, yet most of these systems still require some degree of human supervision. Ideally, future robots should explore unknown environments autonomously and independently, continuously collecting data and learning from this data.
Researchers at Carnegie Mellon University recently created ALAN, a robotic agent that can autonomously explore unfamiliar environments. This robot, introduced in a paper pre-published on arXiv and set to be presented at the International Conference of Robotics and Automation (ICRA 2023), was found to successfully complete tasks in the real-world after a brief number of exploration trials.
“We have been interested in building an AI that learns by setting its own objectives,” Russell Mendonca, one of the researchers who carried out the study, told Tech Xplore. “By not depending on humans for supervision or guidance, such agents can keep learning in new scenarios, driven by their own curiosity. This would enable continual generalization to different domains, and discovery of increasingly complex behavior.”
The robotics group at Carnegie Mellon University had already introduced some autonomous agents that could perform well on new tasks with little or no additional training, including a model trained to play the Mario video-game and a system that could complete multi-stage object manipulation tasks. However, these systems were only trained and tested in simulated environments.
The key objective of the team’s recent study was to create a framework that could be applied to physical robots in the world, improving their ability to explore their surroundings and complete new tasks. ALAN, the system they create, learns to explore its environment autonomously, without receiving rewards or guidance from human agents. Subsequently, it can repurpose what it learned in the past to tackle new tasks or problems.
“ALAN learns a world model in which to plan its actions, and directs itself using environment-centric and agent-centric objectives,” Mendonca explained. “It also reduces the workspace to the area of interest using off the shelf pretrained detectors. After exploration, the robot can stitch the discovered skills to perform single and multi-stage tasks specified via goal images.”
The researchers’ robot features a visual module that can estimate the movements of objects in its surroundings. This module then uses these estimations of how objects have moved to maximize the change in objects and encourage the robot to interact with these objects.
“This is an environment centric signal, since it is not dependent on the agent’s belief,” Mendonca said. “To improve its estimate of the change in objects, ALAN needs to be curious about it. For this, ALAN uses its learned model of the world to identify actions where it is uncertain about the predicted object change, and then executes them in the real world. This agent-centric signal evolves as the robot sees more data.”
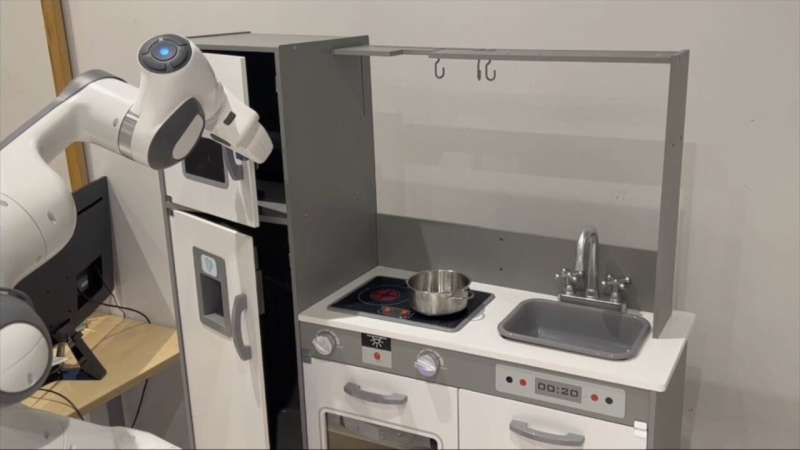
Previously proposed approaches for autonomous robot exploration required large amounts of training data. This prevents or significantly limits their deployment on real robots. In contrast, the learning approach proposed by Mendonca and his colleagues allows the ALAN robot to continuously and autonomously learn to complete tasks as it is exploring their surroundings.
“We show that ALAN can learn how to manipulate objects with only around 100 trajectories in 1–2 hours in two distinct play kitchens, without any rewards,” Mendonca said. “Hence, using visual priors can greatly increase efficiency of robot learning. Scaled up versions of this system that are run in a 24/7 manner will be able to continually acquire new useful skills with minimal human intervention across domains, bringing us closer to general-purpose intelligent robots.”
In initial evaluations, the team’s robot performed remarkably well, as it was able to quickly learn to complete new manipulation tasks without any training or help from human agents. In the future, ALAN and the framework underpinning it could pave the way for the creation of better performing autonomous robotic systems for environment exploration.
“Next we want to study how to utilize other priors to help structure the robot’s behavior, such as videos of humans performing tasks and language descriptions,” Mendonca added. “Systems that can effectively build upon this data will be able to autonomously explore better by operating in structured spaces. Further, we are interested in multi-robot systems that can pool their experience to continually learn.”
More information:
Russell Mendonca et al, ALAN: Autonomously Exploring Robotic Agents in the Real World, arXiv (2023). DOI: 10.48550/arxiv.2302.06604
© 2023 Science X Network
Citation:
A robot that can autonomously explore real-world environments (2023, March 9)
retrieved 9 March 2023
from https://techxplore.com/news/2023-03-robot-autonomously-explore-real-world-environments.html
This document is subject to copyright. Apart from any fair dealing for the purpose of private study or research, no
part may be reproduced without the written permission. The content is provided for information purposes only.
For all the latest Technology News Click Here
For the latest news and updates, follow us on Google News.